If You Can't Take the Heat Map: Benefits & Risks of Releasing Location Datasets
Strava’s location data controversy demonstrates the unique challenges of publicly releasing location datasets (open data), even when the data is aggregated.
This weekend, the Washington Post reported that an interactive “Global Heat Map,” published by fitness data company Strava, had revealed sensitive information about the location and movements of servicemen and women in Iraq, Syria, and other conflict zones. The data in Strava’s Global Heat Map originates from individual users’ fitness tracking data, although the company took steps to de-identify and aggregate the information. The resulting controversy has highlighted some of the serious privacy challenges of publicly releasing open data sets, particularly when they are based on sensitive underlying information (in this case, geo-location).
Until recently, almost all conversations around open data related to the risks of re-identification, or the possibility that individual users might be identified from within an otherwise anonymous dataset. The controversy around Strava demonstrates clearly that risks of open data can go far beyond individual privacy. In addition to the identity of any individual user, location data can also reveal: the existence of – or activities related to – sensitive locations; group mobility patterns; and individual mobility patterns (even if particular people aren’t identified).
As we recommended in our recent privacy assessment for the City of Seattle, companies should thoroughly analyze the range of potential unintended consequences of an open data set, including risks to individual privacy (re-identification), but also including societal risks: quality, fairness, equity, and public trust.
What happened?
Strava is a San Francisco-based company that provides location-based fitness tracking for runners and cyclists (calling itself the “social network for athletes”). Users can download Strava’s free app and use it to directly map their workouts, or pair it with a fitness device, such as a FitBit. In this sense, Strava is very similar to dozens of other popular fitness tracking apps, such as MapMyRun, RunKeeper, or Nike + Run Club.
In providing this service, Strava collects precise location data from users’ smartphones as they run or cycle. Like many fitness apps, Strava makes this data “public” by default, but provides adjustable privacy controls for its community-based features, such as public leaderboards, visibility to nearby runners, and group activities. In fact, Strava deserves some credit for providing more granular privacy controls than most fitness apps — they allow users to selectively hide their activities from others, or to create “Privacy Zones” around their home or office. While there is undoubtedly work to be done to align defaults with users’ expectations (we note, for example, that Strava’s request for access to location data does not mention public sharing, but asks for location “so you can track your activities”), many users of fitness apps will be familiar with this kind of setup, and able to exercise informed privacy choices.
Apart from users’ privacy controls with respect to other athletes, Strava itself maintains users’ historical location data that it collects in the process of providing its fitness tracking. Like nearly all location-based apps, Strava states in its Privacy Policy that it has the right to use de-identified and aggregated location information for its own purposes, including selling or licensing it, or using it for research or commercial purposes. Because de-identified information is considered by some to present fewer privacy risks (or none at all), this is a common statement that can be found in many privacy policies.
Facing Heat for Heat Maps
Beginning in 2017, Strava began publishing an updated “Global Heat Map” of the aggregated jogging and cycling routes of its 27 million users. As far as we can tell, the Heat Map is comprised of the anonymous location data collected and aggregated from all users, even when they have enabled the app’s primary privacy controls vis a vis other athletes — although according to Strava’s support page, the Heat Map excludes “private activities” (activities that users have set to be totally private) and “zones of privacy” (users’ activities within areas that they have specifically submitted to Strava to be excluded, such as home and work addresses).
Per Strava, the aggregated patterns of its users are meant to be used to “improve bike- and pedestrian-friendly infrastructure in your area . . . [including for] departments of transportation and city planning groups to plan, measure and improve infrastructure for bicyclists and pedestrians.”
Strava is not alone in this endeavor — there is a growing industry for location data from a wide variety of sources, including fitness trackers and location-based mobile apps. For example, Uber Movement promises to harness the power of its user base by providing “anonymized data from over two billion trips to help urban planning around the world.” Similarly, many state and local governments are partnering with Waze to share mobility data and reduce traffic.
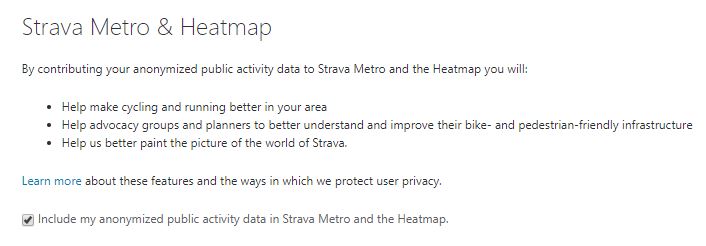
Strava allows users to opt out of contributing to the Global Heat Map, although the option is only available in the online dashboard (not the app).
Despite Strava’s removal of personal information from the data, and their aggregation (to the level of the street grid), careful observers noticed last week that the Heat Map contained location patterns from within U.S. military bases in active conflict zones, including Syria and Afghanistan. While the existence of many of these military installations are already known, others have noted that the Heat Map revealed other “airstrips and base-like shapes in places where neither the American-led military forces nor the Central Intelligence Agency are known to have personnel stations.” (NYTimes)
Perhaps more importantly, the Heat Map reveals mobility patterns between and within military installations that give rise to security and safety concerns. Accordingly, the U.S. coalition against the Islamic State has stated that it is “refining” its existing rules on fitness tracking devices (WP), and a spokesperson for US Central Command has noted that the military is looking “into the implications of the map.” (The Verge)
Addressing Open Data Challenges (Aggregating is Not Always Enough)
While there is certainly an important conversation to be had about individual users having a better understanding of the information they share, it is equally important to consider the responsibilities that are involved with any public release of a large dataset. In Strava’s case, the company was under no obligation to make the data they held available to the public. They did so in order to provide an interesting, useful feature, and perhaps to demonstrate their powerful mapping capabilities. Once they decided to release the data, however, they had a responsibility to thoroughly review it for potential risks (no easy task).
These kinds of challenges are by no means new. As FPF’s Jules Polonetsky, Omer Tene, and Kelsey Finch described in Shades of Grey: Seeing the Full Spectrum of Practical Data De-Identification, some of the most (in)famous examples of “re-identification” arose from the public release of AOL search data, a Massachusetts medical database, Netflix recommendations, and an open genomics database. In each of these cases, “even though administrators had removed any data fields they thought might uniquely identify individuals, researchers . . . unlocked identity by discovering pockets of surprising uniqueness remaining in the data.” Repeatedly, researchers have shown that in a big data world, even mundane data points, such as the battery life remaining on an individual’s phone, can serve as potent identifiers singling out an individual from the crowd.
Until recently, however, almost all conversations around open data related to the risks of re-identification, or the possibility that individual users might be identified from within an otherwise anonymous dataset. However, risks of open data can go far beyond individual privacy. In addition to the identity of any individual user, location data can also reveal:
- individual mobility patterns, including sensitive behavior (Supreme Court Justice Sotomayor wrote in 2012 that location data “generates a precise, comprehensive record of a person’s public movements that reflects a wealth of detail about her familial, political, professional, religious, and sexual associations. . . .”);
- group mobility patterns (in the case of Strava, it was quickly noticed that supply routes and exercise habits of overseas servicemen and women could be detected in the data, facts which hold implications for safety and security); and
- existence or activities related to sensitive location (while some locations, such as abortion clinics or mosques, may only be sensitive in their relation to individual visitors, other locations, such as secret military installations, may be sensitive in their very existence).
In our recent work with the City of Seattle, we explored risks related to Seattle’s Open Data Program, in which the local government releases a variety of useful data about city activities (such as 911 calls, building permits, traffic flow counts, public parks and trails, and food bank locations). While Seattle’s civic data is “open by preference,” the city recognized that “some data elements, if released, could cause privacy harms, put critical infrastructure at risk, or put public safety personnel and initiatives at risk.”
In our Open Data Privacy Risk Assessment, we recommended that any entity deciding whether (or how) to publicly release a large dataset should undergo a privacy benefit-risk assessment. Specifically, we recommended a thorough analysis of several areas of risk:
#1. Re-identification. As we describe in the Open Data Risk Assessment, one of the principal risks of open datasets is the possibility that the data might reveal sensitive information about a specific individual. Once information has been published publicly, it is difficult or impossible to retract — and as a result, (per our Program Maturity Assessment) data holders should:
- Utilize technical, legal, and administrative safeguards to reduce re-identification risk;
- Have access to disclosure control experts to evaluate re-identification risk;
- Use appropriate tools to de-identify unstructured or dynamic data types (e.g., geographic, video, audio, free text, real time sensor data);
- Have policies and procedures for evaluating re-identification risk across databases (e.g., risk created by intersection of databases; county, state, or federal open databases; and commercial databases).
Although initial reports indicated that the Strava Heat Map did not reveal individual information, combining the aggregate Heat Map data and the Strava’s leaderboards can potentially reveal individual users and the details of their top runs. Reportedly, a computer scientist has already developed a programmatic method for accessing individual names directly from the Heat Map datasets without relying on outside information.
#2. Data Quality. If data is going to be released publicly, the data should be (as far as possible) accurate, complete, and current. Although this is certainly more important in the context of government-held data, it is applicable to companies like Strava in the sense that the data should reliably inform future decisions. Companies can take steps to check for inaccurate or outdated information, and institute procedures for individuals to submit corrections as appropriate.
#3. Equity and Fairness. Another key aspect of open data — equally applicable to private datasets as to government-held datasets — is equity and fairness. If data is going to be released publicly to be used by others, it should be collected fairly and assessed for its representativeness. For example, Strava permits its users to opt out of contributing to the open dataset (although many may have been unaware), an important aspect of fairness.
Equally, though, datasets should be representative. In his comments to the Washington Post, the Australian researcher who first noticed problems with Strava’s Heat Map mentioned that his father had suggested he check it out as “a map of rich white people.” Although the comment was likely offhand, the issues are serious — particularly in “smart” systems that use algorithmic decision-making, bad data can lead to bad policies. For example, both predictive policing and criminal sentencing have repeatedly demonstrated racial bias in both the inputs (historic arrest and recidivism data) and their outputs, leading to new forms of institutional racial profiling and discrimination.
#4. Public Trust. Although we might typically think of “public” trust as a government issue, trust is critical in the private sector as well. As we have seen in the resulting discussions of Strava and the collection of location data from fitness trackers, there is concern both in the military and amongst average consumers around the use of connected devices. Particularly in the absence of baseline privacy legislation, trust is critical for the growth of new technologies, including Internet of Things (IoT) devices, fitness trackers, Smart Cities, and connected cars.
Conclusion
Beyond individual privacy, the Strava Heat Map demonstrates that there are societal risks that inhere in sensitive datasets. In particular, geo-location data can reveal individual and group patterns of movement, as well as information related to sensitive locations that must be taken into account. As technology advances and we address the challenges of connected Internet of Things (IoT) devices in our homes, on our bodies, and embedded in our cities, it is more important than ever to address the privacy challenges of location and open data.