Unfairness By Algorithm: Distilling the Harms of Automated Decision-Making
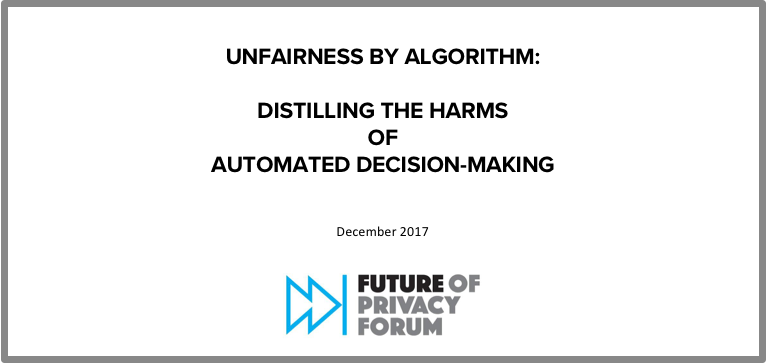
Analysis of personal data can be used to improve services, advance research, and combat discrimination. However, such analysis can also create valid concerns about differential treatment of individuals or harmful impacts on vulnerable communities. These concerns can be amplified when automated decision-making uses sensitive data (such as race, gender, or familial status), impacts protected classes, or affects individuals’ eligibility for housing, employment, or other core services. When seeking to identify harms, it is important to appreciate the context of interactions between individuals, companies, and governments—including the benefits provided by automated decision-making frameworks, and the fallibility of human decision-making.
Recent discussions have highlighted legal and ethical issues raised by the use of sensitive data for hiring, policing, benefits determinations, marketing, and other purposes. These conversations can become mired in definitional challenges that make progress towards solutions difficult. There are few easy ways to navigate these issues, but if stakeholders hold frank discussions, we can do more to promote fairness, encourage responsible data use, and combat discrimination.
To facilitate these discussions, the Future of Privacy Forum (FPF) attempted to identify, articulate, and categorize the types of harm that may result from automated decision-making. To inform this effort, FPF reviewed leading books, articles, and advocacy pieces on the topic of algorithmic discrimination. We distilled both the harms and potential mitigation strategies identified in the literature into two charts. We hope you will suggest revisions, identify challenges, and help improve the document by contacting [email protected]. In addition to presenting this document for consideration for the FTC Informational Injury workshop, we anticipate it will be useful in assessing fairness, transparency and accountability for artificial intelligence, as well as methodologies to assess impacts on rights and freedoms under the EU General Data Protection Regulation.
The Chart of Potential Harms from Automated Decision-Making
This chart groups the harms identified in the literature into four broad “buckets”—loss of opportunity, economic loss, social detriment, and loss of liberty—to depict the various spheres of life where automated decision-making can cause injury. It also notes whether each harm manifests for individuals or collectives, and as illegal or simply unfair.
We hope that by identifying and categorizing the harms, we can begin a process that will empower those seeking solutions to mitigate these harms. We believe that a more clear articulation of harms will help focus attention and energy on potential mitigation strategies that can reduce the risks of algorithmic discrimination. We attempted to include all harms articulated in the literature in this chart; we do not presume to establish which harms pose greater or lesser risks to individuals or society.
The Chart of Potential Mitigation Sets
This chart uses FPF’s taxonomy to further categorize harms into groups that are sufficiently similar to each other that they could be amenable to the same mitigation strategies.
Attempts to solve or prevent this broad swath of harms will require a range of tools and perspectives. Such attempts benefit by further categorization of the identified harms, into five groups of similar harms. These groups include: (1) individual harms that are illegal; (2) individual harms that are simply unfair, but have a corresponding illegal analog; (3) collective/societal harms that have a corresponding individual illegal analog; (4) individual harms that are unfair and lack a corresponding illegal analog; and (5) collective/societal harms that lack a corresponding individual illegal analog. The chart includes a description of the mitigation strategies that are best positioned to address each group of harms.
There is ample debate about whether the lawful decisions included in this chart are fair, unfair, ethical, or unethical. Absent societal consensus, these harms may not be ripe for legal remedies.