Automated Decision-Making Systems: Considerations for State Policymakers
In legislatures across the United States, state lawmakers are introducing proposals to govern the uses of automated decision-making systems (ADS) in record numbers. In contrast to comprehensive privacy bills that would regulate collection and use of personal information, automated decision-making system (ADS) bills in 2021 specifically seek to address increasing concerns about racial bias or unfair outcomes in automated decisions that impact consumers, including housing, insurance, financial, or governmental decisions.
So far, ADS bills have taken a range of approaches, with most prioritizing restrictions on government use and procurement of ADS (Maryland HB 1323); requiring inventories of government ADSs currently in use (Vermont H 0236); impact assessments for procurement (CA AB-13); external audits (New York A6042); or outright prohibitions on the procurement of certain types of unfair ADS (Washington SB 5116). A handful of others would seek to regulate commercial actors, including in insurance decisions (Colorado SB 169), consumer finance (New Jersey S1943), or the use of automated decision-making in employment or hiring decisions (Illinois HB 0053, New York A7244).
At a high level, each of these bills share similar characteristics. Each proposes general definitions and general solutions that cover specific, complex tools used in areas as varied as traffic forecasting and employment screening. But the bills are not consistent with regard to requirements and obligations. For example, among the bills that would require impact assessments, some require impact assessments universally for all ADS in use by government agencies, others would require impact assessments only for specifically risky uses of ADS.
As states evaluate possible regulatory approaches, lawmakers should: (1) avoid a “one size fits all” approach to defining automated decision-making by clearly defining the particular systems of concern; (2) consult with experts in governmental, evidence-based policymaking; (3) ensure that impact assessments and disclosures of risk meet the needs of their intended audiences; (4) look to existing law and guidance from other state, federal, and international jurisdictions; and (5) ensure appropriate timelines for technical and legal compliance, including time for building capacity and attracting qualified experts.
1. Avoid “one size fits all” solutions by clearly identifying the automated decision-making systems of concern.
An important first step to the regulation of automated decision-making systems (“ADS”) is to identify the scope of systems that are of concern. Many lawmakers have indicated that they are seeking to address automated decisions such as those that use consumer data to create “risk scores,” creditworthiness profiles, or other kinds of profiles that materially impact our lives and involve the potential for systematic bias against categories of people. But, the wealth of possible forms of ADS and the many settings for their use can make defining these systems in legislation very challenging.
Automated systems are present in almost all walks of modern life, from managing wastewater treatment facilities to performing basic tasks such as operating traffic signals. ADS can automate the processing of personal data, administrative data, or myriad forms of other data, through the use of tools ranging in complexity from simple spreadsheet formulas, to advanced statistical modeling, rules-based artificial intelligence, or machine learning. In an effort to navigate this complexity, it can be tempting to draft very general definitions of ADS. However, these definitions risk being overbroad and capturing ADS systems that are not truly of concern — i.e. because they do not impact people or carry out significant decision-making.
For example, a definition such as “a computational process, including one derived from machine learning, statistics, or other data processing or artificial intelligence techniques, that makes a decision or facilitates human decision-making” (New Jersey S1943) would likely include a wide range of traditional statistical data processing, such as estimating average number of vehicles per hour on a highway to facilitate automatic lane closures in intelligent traffic systems. This would place an additional, significant requirement for conducting complex impact assessments for many of the tools behind established operational processes. In contrast, California’s AB-13 takes a more tailored approach, aiming to regulate “high-risk application[s]” of algorithms that involve “a score, classification, recommendation, or other simplified output,” that support or replace human decision-making, in situations that “materially impact a person” (12115(a)&(b)).
In general, compliance-heavy requirements or prohibitions on certain practices may be appropriate only for some high-risk systems. The same requirements would be overly prescriptive or infeasible for systems powering ordinary, operational decision-making. Successfully distinguishing between high-risk use cases and those without significant, personal impact will be crucial to crafting tailored legislation that addresses the targeted, unfair outcomes without overburdening other applications.
Lawmakers should ask questions such as:
- Who owns or is responsible for the ADS? Is the system being used by government decision-makers, commercial actors, or both (private vendors contracted by government agencies)? The relevant “owner” of a system may determine the right balance of transparency, accountability, and access to underlying data necessary to accomplish the legislative goals.
- What kind of data is involved? Many systems use a wide range of data that may or may not include personal information (information related to reasonably identifiable individuals), and may or may not include “sensitive data” (personal data that reveals information about race, religion, health conditions, or other highly personal information). In some cases, non-sensitive data can act as a “proxy” for sensitive information (such as the use of zip code as a proxy for race). Data may also be obtained from sources of varying quality, accuracy, or ethical collection, for example: public records, government collection, regulated commercial sectors (banks or credit agencies), commercial data brokers, or other commercial sources.
- Who is impacted by the decision-making? Does the decision-making impact individuals, groups of individuals, or neither? Is there a possibility for disparate impact in who is affected, i.e. that certain races, genders, income levels, or other categories of people will be impacted differently or worse than others?
- Is the decision-making legally significant? In most cases, our tolerance for automated decision-making depends on the decision being made. Some decisions are commonplace or operational, such as automated electrical grid management. Other decisions are so relevant to our individual lives and autonomy that use of automated systems in this context demands greater transparency, human involvement, or even auditing such as: financial opportunities, housing, lending, educational opportunities, or employment. Still other decisions may be in a “grey area”: for example, automated delivery of online advertisements is common, but questions about algorithmic bias in ad quality or who sees certain types of ads (e.g. ads for particular jobs) are leading to increasing scrutiny.
- Does the system assist human decision-making or replace it? Some systems replace human decision-making entirely, such as when a system generates an automated approval or denial of a financial opportunity that occurs without human review. Other systems assist human decision-makers by generating outputs such as scores or classifications that allow decision-makers to complete tasks, such as grading a test or diagnosing a health condition.
- When do “meaningful changes” occur? Many legislative efforts seek to trigger requirements for new or updated impact assessments when ADSs change, or “meaningfully change.” For such requirements, lawmakers should establish clear criteria for what constitutes a “meaningful change.” For example, machine learning systems that adapt based upon a stream of sensor or customer data change constantly, whether by changing the weights attached to features or by eliminating features. Whether adaptations made as a consequence of typical machine learning operations constitute meaningful changes is an important question best poised to be answered in ways specific to each learning and adapting system. The velocity and variety of changes to ADS driven by machine learning may require other forms of ongoing assessment to identify abnormalities or potential harms as they arise.
These questions can help guide legislative definitions and scope. A “one size fits all” solution not only risks creating burdensome requirements in situations where they are not needed, but is also less likely to ensure stronger requirements in situations where they are needed — leaving potentially biased algorithms to operate without sufficient review or standards to address resulting outcomes that are biased or unfair. An appropriate definition is a critical first step for effective regulation.
2. Consult with experts in governmental, evidence-based policymaking.
Evidence-based policymaking legislation, popular in the late 1990s and early 2000s, required states to construct systems to eradicate human bias by employing data-driven practices for key areas of state decision-making, such as criminal justice, student achievement predictions, and even land use planning. For example, as defined by the National Institute of Corrections, the vision for implementing evidence based practice in community corrections is “to build learning organizations that reduce recidivism through systematic integration of evidence-based principles in collaboration with community and justice partners” (see resources at the Judicial Council of California 2021). The areas chosen for application of evidence-based policymaking are presently causing high degrees of concern about applications of ADS as the mechanisms for ensuring use of evidence and elimination of subjectivity. Examining the goals envisioned in evidence-based policymaking legislation may clarify whether ADS are appropriate tools for satisfying those goals.
In addition to consulting the policies encouraging evidence-based making in order to identify the goals for automated decision-making systems (ADSs) the evidence-based research findings reviewed to support this legislation can also direct legislators to contextually relevant, expert, sources of data that should be incorporated into ADS or into the evaluation of ADS. Likewise, legislators should reflect on the challenges to implementation of effective evidence-based decision-making, such as unclear definitions, poor data quality, challenges to statistical modelling, and a lack of interoperability of public data sources, as these challenges are similar to those complicating use of ADS.
3. Ensure that impact assessments and disclosures of risk meet the needs of their intended audiences.
Most ADS legislative efforts aim to increase transparency or accountability through various forms of mandated notices, disclosures, data protection impact assessments, or other risk assessments and mitigation strategies. These requirements serve multiple, important goals, including helping regulators understand data processing, and increasing internal accountability through greater process documentation. In addition, public disclosures of risk assessments benefit a wide range of stakeholders, including: the public, consumers, businesses, regulators, watchdogs, technologists, and academic researchers.
Given the needs of different audiences and users of such information, lawmakers should ensure that impact assessments and mandated disclosures are leveraged effectively to support the goals of the legislation. For example, where legislators intend to improve equity of outcomes between groups, they should include legislative support for tools to improve communication to these groups and to support incorporation of these groups into technical communities. Where sponsors of ADS bills intend to increase public awareness of automated decision-making in particular contexts, legislation should require and fund consumer education that is easy to understand, available in multiple languages, and accessible to broad audiences. In contrast, if the goal is to increase regulator accountability and technical enforcement, legislation might mandate more detailed or technical disclosures be provided non-publicly or upon request to government agencies.
The National Institutes of Standards and Technology (NIST) has offered recent guidance on explainability in artificial intelligence that might serve as a helpful model for ensuring that impact assessments are useful for the multiple audiences they may serve. The NIST draft guidelines suggest four principles for explainability for audience sensitive, purpose driven, ADS assessment tools: (1) Systems offer accompanying evidence or reason(s) for all outputs; (2) Systems provide explanations that are understandable to individual users; (3) The explanation correctly reflects the system’s process for generating the output; and (4) The system only operates under conditions for which it was designed or when the system reaches a sufficient confidence in its output (p.2). These four principles shape the types of explanations needed to ensure confidence in algorithmic or automated decision-making systems (ADSs), such as explanations for user benefit, for social acceptance, for regulatory and compliance purposes, for system development, and for owner benefit (p. 4-5).
Similarly, the European Commission’s Guidelines on Automated Individual Decision-Making and Profiling provides recommendations for complying with the GDPR’s requirement that individual users be given “meaningful information about the logic involved.” Rather than requiring a complex explanation or exposure of the algorithmic code, the Commission explains that a controller should find simple ways to tell the data subject the rationale behind, or the criteria relied upon to reach a decision. This may include which characteristics are considered to make a decision, the source of the information, and its relevance. It should not be overly technical, but sufficiently comprehensive for a consumer to understand the reason for the decision.
Regardless of the audience, mandated disclosures should be used cautiously as, especially when made public, such disclosures can also create certain risks, such as opportunities for data breaches, exfiltration of intellectual property (IP), or even attacks on the algorithmic system which could identify individuals or cause the systems to behave in unintended ways.
4. Look to existing law and guidance from other state, federal, and international jurisdictions.
Although US lawmakers have specific goals, needs, and concerns driving legislation in their jurisdictions, there are clear lessons to be learned from other regimes with respect to automated decision-making. Most significantly, there has been a growing, active wave of legal and technical guidance in the European Union in recent years regarding profiling and automated decision-making, following the passage of the GDPR. Lawmakers may also seek to ensure interoperability with the newly passed California Privacy Rights Act (CPRA) or Virginia Consumer Data Protection Act (VA-CDPA), both of which create requirements that impact automated decision-making, including profiling. Finally, the Federal Trade Commission enforces a number of laws that could be harnessed to address concerns about biased or unfair decision-making. Of note, Singapore is also a leader in this space, launching their Model AI Governance Framework in 2019. It is useful to understand the advantages or limitations of each model and to recognize the practical challenges of adapting systems for each jurisdiction.
General Data Protection Regulation (GDPR)
The EU General Data Protection Regulation (GDPR) broadly regulates public and private collection of personal information. This includes a requirement that all data processing be fair (Art. 5(1)(a)). The GDPR also creates heightened safeguards specifically for high risk automated processing that impact individuals, especially with respect to decisions that produce legal, or other significant, effects concerning individuals. These safeguards include organizational responsibilities (data protection impact assessments); and individual empowerment provisions (disclosures, and the right not to be subject to certain kinds of decisions based solely on automated processing).
- Organizational Responsibilities. Data protection impact assessments (DPIAs) required under the GDPR for “high risk” processing activities, must include a systematic description of the envisaged processing operations and the purposes of the processing, an assessment of the necessity and proportionality of the processing operations in relation to the purposes, an assessment of the risks to the rights and freedoms of data subjects, and measures envisaged to address the risks, including safeguards, security measures and mechanisms to ensure the protection of personal data. Recital 75 of the GDPR, which details the Art. 35 DPIA requirements, provides details about the nature of the data processing risks intended to be covered. In addition the GDPR requires all automated processing to incorporate technical and organizational measures to implement data protection by design principles (Art. 25).
- Individual Control. In addition to providing organizational responsibilities such as data protection impact assessments (DPIAs), the GDPR also requires controllers to provide data subjects with information relating to their automated processing activities (Art. 13 & 14). In particular, controllers must disclose the existence of automated decision-making, including profiling, meaningful information about the logic involved, and the significance and envisaged consequences of processing for the data subject. These disclosures are required when personal data is collected from a data subject, and also when personal data is not obtained from a data subject. In addition, the GDPR creates the right for an individual not to be subject to decisions based solely on automated processing which produce legal, or similarly significant, effects concerning an individual (Art. 22). Suitable measures to safeguard the data subject’s rights, freedoms, and legitimate interests include the rights for an individual to: (1) obtain human intervention on the part of the controller (human in the loop), (2) express their point of view, and (3) contest a decision.
California Privacy Rights Act (CRPA)
The California Privacy Rights Act (CPRA), passed via Ballot Initiative in 2020, expands on the California Consumer Privacy Act (CCPA)’s requirements that businesses comply with consumer requests to access, delete, and opt-out of the sale of consumer data.
While the CPRA does not create any direct consumer rights or organizational responsibilities with respect to automated decision-making, its consumer access rights includes access to information about “inferences drawn . . . to create a profile” (Sec. 1798.140(v)(1)(K)) and most likely information about the use of the consumer’s data for automated decision-making.
Notably, the CPRA added a new definition of “profiling” to the CCPA, while authorizing the new California oversight agency to engage in rulemaking. In alignment with the GDPR, the CPRA defines “profiling” as “any form of automated processing of personal Information . . . to evaluate certain personal aspects relating to a natural person, and in particular to analyze or predict aspects concerning that natural person’s performance at work, economic situation, health, personal preferences, interests, reliability, behavior, location or movements” (1798.140(z)).
The CPRA authorizes the new California Privacy Protection Agency to issue regulations governing automated decision-making, including “governing access and opt‐out rights with respect to businesses’ use of [ADS], including profiling and requiring businesses’ response to access requests to include meaningful information about the logic involved in such decision-making processes, as well as a description of the likely outcome of the process with respect to the consumer.” (1798.185(a)(16)). Notably, this language lacks the GDPR’s “legal or similarly significant” caveat, meaning that the CPRA requirements around access and opt-outs may extend to processing activities such as targeted advertising based on profiling.
Virginia Consumer Data Protection Act (VA-CDPA)
The Virginia Consumer Data Protection Act (VA-CDPA), which passed in 2021 in Virginia and will come into effect in 2023, takes an approach towards automated decision-making inspired by both the GDPR and CPRA.
First, its definition of “profiling” aligns with that of the GDPR and CPRA (§ 59.1-571). Second, it imposes a responsibility upon data controllers to conduct data protection impact assessments (DPIAs) for high risk profiling activities (§ 59.1-576). Third, it creates a right for individuals to opt out of having their personal data processed for the purpose of profiling in the furtherance of decisions that produce legal or similarly significant effects concerning the consumer (§ 59.1-573(5)).
- Organizational Responsibilities. The VA-CDPA requires data controllers to conduct and document data protection impact assessments (DPIAs) for “profiling” that creates a “reasonably foreseeable risk of (i) unfair or deceptive treatment of, or unlawful disparate impact on, consumers; (ii) financial, physical, or reputational injury to consumers; (iii) a physical or other intrusion upon the solitude or seclusion, or the private affairs or concerns, of consumers, where such intrusion would be offensive to a reasonable person; or (iv) other substantial injury to consumers.” These DPIA’s are required to identify and weigh the benefits against the risks that may flow from the processing, as mitigated by safeguards employed to reduce such risks. They are not intended to be made public or provided to consumers. Instead, these confidential documents must be made available to the State Attorney General upon request, pursuant to an investigative civil demand.
- Individual Control. The VA-CDPA grants consumers the right to submit an authenticated request to opt-out of the processing of personal data for purposes of profiling “in the furtherance of decisions that produce legal or similarly significant effects concerning the consumer,” which is defined as “a decision made by the controller that results in the provision or denial by the controller of financial and lending services, housing, insurance, education enrollment, criminal justice, employment opportunities, health care services, or access to basic necessities, such as food and water.”
The FTC Act and broadly applicable consumer protection laws
Finally, a range of federal consumer protection and sectoral laws already apply to many businesses’ uses of automated decision-making systems. The Federal Trade Commission (FTC) enforces long-standing consumer protection laws prohibiting “unfair” and “deceptive” trade practices, including the FTC Act. As recently as April 2021, the FTC warned businesses of the potential for enforcement actions for biased and unfair outcomes in AI, specifically noting that the “sale or use of – for example – racially biased algorithms” would violate Section 5 of the FTC Act.
The FTC also noted its decades of experience enforcing other federal laws that are applicable to certain uses of AI and automated decisions, including the Fair Credit Reporting Act (if an algorithm is used to deny people employment, housing, credit, insurance, or other benefits), and the Equal Credit Opportunity Act (making it “illegal for a company to use a biased algorithm that results in credit discrimination on the basis of race, color, religion, national origin, sex, marital status, age, or because a person receives public assistance”).
Comparison chart:
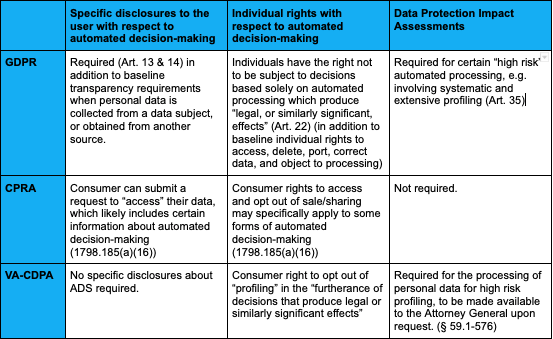
5. Ensure appropriate timelines for technical and legal compliance, including building capacity and attracting qualified experts.
In general, timelines for government agencies and companies to comply with the law should be appropriate to the complexity of the systems that will be needed to review for impact. Many government offices may not be aware that the systems they use every day to improve throughput, efficiency, and effective program monitoring may constitute “automated decision-making.” For example, organizations using Customer Relations Management (CRM) software from large vendors may be using predictive and profiling systems built into that software. Also, governmental offices suffer from siloed procurement and development strategies and may have built or purchased overlapping ADS to serve specific, sometimes narrow, needs.
Lack of government funding, modernization, or resources to address the complexity of the systems themselves, and the lack of prior requirements for tracking automated systems in contracts or procurement decisions, means that many agencies will not readily have access to technical information on all systems in use. Automated decision-making systems (ADSs) have been shown to suffer from technological debt, opaque and incomplete technical documentation, or are dependent on smaller automated systems that can only be discovered through careful review of source code and complex information architectures.
Challenges such as these were highlighted during 2020 as a result of the COVID-19 pandemic, which prompted millions to pursue temporary unemployment benefits. When applications for unemployment benefits surged, some state unemployment agencies discovered that their programs were written in the infrequently used programming language, COBOL. Many resource-strapped agencies were using stop-gap code, intended for temporary use, to translate COBOL into more contemporary coding languages. As a result, many agencies lacked programming experts and capacity to efficiently process the influx of claims. Regulators should ensure that offices have time, personnel, and funding to undertake the digital archaeology necessary to reveal the many layers of ADSs used today.
Finally, lawmakers should not overlook the challenges of identifying and attracting qualified technical and legal experts. For example, many legislative efforts envision a new or expanded government oversight office with the responsibility to review automated impact assessments. Not only will the personnel needed for these offices need to be able to meaningfully interpret algorithmic impact assessments, they will need to do so in an environment of high sensitivity, publicity, and technological change. As observed in many state and federal bills calling for STEM and AI workforce development, the talent pipeline is limited and legislatures should address the challenges of attracting appropriate talent as a key component of these bills. Likewise, identifying appropriate expectations of performance, including ethical performance, for ADS review staff will take time, resources, and collaboration with new actors, such as the National Society of Professional Engineers, whose code of conduct governs many working in fields responsible for designing or using ADS.
What’s Next for Automated Decision System Regulation?
States are continuing to take up the challenge of regulating these complex and pervasive systems. To ensure that these proposals achieve their intended goals, legislators must address the ongoing issues of definition, scope, audience, timelines and resources, and mitigating unintended consequences. More broadly, legislation should help motivate more challenging public conversations about evaluating the benefits and risks of using ADS as well as the social and community goals for regulating these systems.
At the highest level, legislatures should bear in mind that ADS are engineered systems or products that are subject to product regulations and ethical standards for those building products. In addition to existing laws and guidance, legislators can consult the norms of engineering ethics, such as the NSPE’s code of ethics, which requires that engineers ensure their products are designed so as to protect as paramount the safety, health and welfare of the public. Stakeholder engagement, including with consumers, technologists, and the academic community, is imperative to ensuring that legislation is effective.
Additional Materials:
- Future of Privacy Forum, “FPF Testifies On Automated Decision System Legislation In California” (Apr. 2021)
- Future of Privacy Forum, Verbal Testimony of Dr. Sara Jordan Before the Vermont House Committee on Energy and Technology (Apr. 2021)
- Future of Privacy Forum, “The Spectrum of Artificial Intelligence – An Infographical Tool” (Jan. 2021)
- Future of Privacy Forum, “Ten Questions on AI Risk” (July, 2020)
- Future of Privacy Forum, “Unfairness by Algorithm: Distilling the Harms of Automated Decision-Making” (Dec. 2017)
- Federal Trade Commission, “Aiming for truth, fairness, and equity in your company’s use of AI” (Apr. 2021)
- Federal Trade Commission, “Using Artificial Intelligence and Algorithms” (Apr. 2020)
- Federal Trade Commission, “Big Data: A Tool for Inclusion or Exclusion? Understanding the Issues (FTC Report)” (Jan. 2016)
- European Data Protection Board, “Guidelines on Automated individual decision-making and Profiling for the purposes of Regulation 2016/679” (Feb. 2018)
- European Commission, “Proposal for a Regulation on a European approach for Artificial Intelligence” (Apr. 2021)